One Armed Bandit Slot Machine Odds
- One Armed Bandit Slot Machine Odds Win
- One Armed Bandit Slot Machine Odds Free Play
- One Armed Bandit Slot Machine Odds Against
- One Armed Bandit Slot Machine Odds Free
Slot Machine Money Box one arm bandit reels fruit. Slot Machine Money Box - one arm bandit money or parcelink selling other items please take a look many thanks. This original as found jubilee riviera deci coin one arm bandit has been in dry storage for many years and is in not working condition. The one-armed bandit slot machine, also known as the classic slot machine, features one payline and three reels. You can bet the minimum or the maximum, and you only win if the reels form a specific line. Fruit machines are popular machines in parts of Britain and feature hold buttons and buttons that let you earn a bonus for playing. Step up to the ‘one-armed bandit’ – the CLASSIC casino slot machines - just like in old Vegas, anticipating those triple blazing sevens, the jackpot, the lights, the noise, the THRILL! Blazing 7s Classic Casino brings you the best classic slots to play straight from the heart of Vegas - Enjoy free slots with bonus rounds! Define one-armed bandits. One-armed bandits synonyms, one-armed bandits pronunciation, one-armed bandits translation, English dictionary definition of one-armed bandits. A slot machine for gambling operated by pulling a lever on the side. Or n a fruit machine operated by pulling down a lever at one side n. Made Out Like a Bandit Meaning. Definition: To get a large amount of riches or valuable things. Origin of Made Out Like a Bandit. The bandit is this phrase doesn’t refer to an outlaw or robber but to the one-armed bandit of gambling, i.e., a slot machine. Slot machines are known for their terrible odds, so if you were to make out like the slot machine after a weekend at the casino, you. Different machines offer different odds of success, and this book explains how to identify the best machines to play in the casino to guarantee walking out a winner. Whether you're a longtime fan of the one-armed bandit or brand new to slot machines, 'Slots Conquest' will change your approach to slot machines instantly. Multi-Armed Bandit Problem. One of the classical problems in RL is the tension between exploration and exploitation. Slot machines, often referred to as “one-armed bandits” are the inspiration for this problem. A bank of slot machines then creates “multi-armed bandit.” Each of these slot machine has a probability of paying out a jackpot.
The First Ever Pokie Slot Machine
Back in 1891 when poker had become fashionable in America, two enterprising New Yorkers Sittman and Pitt devised a poker machine for a solo player. These early pokie machines were very different to the more exciting online pokie games that we see today! The Sittman and Pitt machines featured a glass panel behind which were five reels, each having 10 playing cards mounted on it. There were no side levers on these machines so instead, when you'd put in a coin, you pressed a plunger down. This spun the reels and the cards flipped over.
The mechanism was delightfully simple but the machine itself didn't pay out (in most states a cash prize would not have been allowed). However the machines were almost always located in bars, so if you got a winning poker hand, the bartender would pass over a prize. These days it's much more convenient to play similar games on the ever popular Aristocrat pokie machines. You'll also find All Online Slots Games on https://davedealer.com/games/slots to play for free or for real money.
On the pictured machine, the best hand (a royal flush) would win you 100 cigars! The smallest prize was a single cigar, awarded for a pair of kings or aces.
Of course, five reels with ten cards only used 50 cards in the deck. To reduce the chances of a royal flush, the two unused cards were generally high value, often a ten and a jack of different suits. This would mean that out of the four royal flushes, only two were possible. On a fair machine, the chances of getting one of these two royal flushes would have been 1 in 50,000.
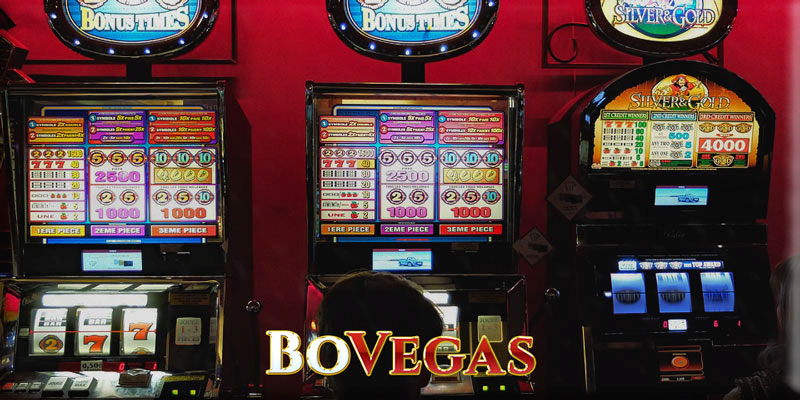
The percentage of wins that any machine allowed could further be controlled by changing the cards around on the reels. An unscrupulous bar owner might have arranged for all four aces to be on the same reel, so eliminating the chance of two, three or four aces coming up. However this would have been extremely foolish as the customers would be sure to notice! Luckily this doesn't happen when playing the modern-dayonline pokies.
-->November 2018
Volume 33 Number 11
By Frank La
In last month’s column, I explored a few basic concepts of reinforcement learning (RL), trying both a strictly random approach to navigating a simple environment and then implementing a Q-Table to remember both past actions and which actions led to which rewards. In the demo, an agent working randomly was able to reach the goal state approximately 1 percent of the time and roughly half the time when using a Q-Table to remember previous actions. However, this experiment only scratched the surface of the promising and expanding field of RL.
Recall that in the previous column (msdn.com/magazine/mt830356), an RL problem space consists of an environment, an agent, actions, states and rewards. An agent examines the state of an environment and takes an action. The action then changes the state of the agent and/or environment. The agent receives a reward and examines the updated state of its environment. The cycle then restarts and runs for a number of iterations until the agent succeeds or fails at a predefined goal. When an agent succeeds or fails, the simulation ends. With a Q-table, an agent remembers which actions yielded positive rewards and references it when making decisions in subsequent simulations.
Multi-Armed Bandit Problem
One of the classical problems in RL is the tension between exploration and exploitation. Slot machines, often referred to as “one-armed bandits” are the inspiration for this problem. A bank of slot machines then creates “multi-armed bandit.” Each of these slot machine has a probability of paying out a jackpot or not. The probability of each turn resulting in a jackpot may be represented as P, and the probability of not paying out is 1 – P. If a machine has a jackpot probability (JP) of .5, then each pull of the lever has an equal chance of winning or losing. Conversely, a machine with a JP of 0.1 would yield a losing result 90 percent of the time.
Now, imagine a bank of five slot machines and the player (or agent) has a goal to maximize winnings and minimize losses. With no foreknowledge of any of the machines’ jackpot probability (JP), the agent must take some risks at first. With the first pull of the lever, the agent wins and receives a payout. However, subsequent tries reveal that this machine pays out about half of the time, a JP of .54. As slot machines go, this is quite generous. The agent must decide if it should exploit the current known resource or explore a new machine. If the probability of the first slot machine paying out is this generous, is it worth trying any of the machines in the bank to see if their payout chances are better?
The best way to further explore this problem space is with some Python code in a Jupyter notebook. Create a Python 3 notebook on your preferred platform. I covered Jupyter notebooks in a previous article (msdn.com/magazine/mt829269). Create an empty cell and enter the following code and execute the cell.
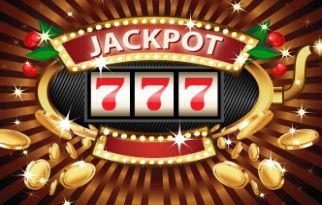
The output should read and show a plot of the values, as shown in Figure 1.
Figure 1 Jackpot Probabilities of the Five Slot Machines
The code creates an array of JP values for a series of five slot machines ranging from 0.004 to 0.844. However, the first machine the agent tried, while generous, is not the best. Clearly, the fourth slot machine with an 84.4 percent payout rate is the best paying machine in the environment. It is also worth noting that the final slot machine has the worst odds of paying out a jackpot. Remember that the agent has no prior knowledge of the payout rates and it must discover them on its own. Had the agent stayed on the first machine, choosing exploitation over exploration, the agent would never have found the best paying slot machine.
To represent what the agent knows at the start of a simulation, add the following code to a new cell:
This creates an array of zeros, meaning that the agent assumes that the JP of each slot machine is zero. While this may not be the best initial value in all cases, it will suffice for our purposes here. To create a simulation of a slot machine, add the following code to a new cell and execute it:
This code snippet simulates a slot machine paying out a reward of 10 if the machine pays out and a negative reward of -1 if the machine does not. Odds of a payout are based on the likelihood defined in the JPs numpy array. To test the code, enter the following Python code into a new cell and execute:
This code pits the best performing machine against the worst performing machine. As this is all based on chance, there’s no guarantee of the output results. The results should reflect that, with a majority of 10 values for machine 4 and nearly all -1 values for machine 5. With the simulated slot machine code behaving as expected, it’s now time to examine a common algorithm in RL: Epsilon Greedy.
The Epsilon Greedy Algorithm
The core dilemma the agent faces here is whether to prioritize greed, the desire to exploit a known resource, or curiosity, the desire to explore other slot machines in the hopes of a better chance of rewards. One of the simplest algorithms for solving this dilemma is known as the Epsilon Greedy algorithm, where the agent chooses at random between using the slot machine with the best odds of payout observed thus far, or trying out another machine in the hopes that it may provide a better payout. With a low value of Epsilon, this algorithm follows the greedy algorithm, but will occasionally try another slot machine. For instance, if the Epsilon value is .1, the algorithm will opt to exploit 90 percent of the time and explore only 10 percent of the time. Typically, default values of Epsilon tend to fall between .05 and .1. In short, the agent will primarily play the best slot machine discovered that it knows of and sometimes try a new machine. Remember that each pull of the lever comes at a cost and the agent doesn’t know what we know: that slot 4 pays out the best.
This underscores the notion of RL. The agent knows nothing about the environment initially, so it needs to first explore, then exploit later. Learning continues throughout the entire process. Essentially, this is the notion of delayed gratification, and it’s in the agent’s best interest not to be totally greedy so it leaves some room for exploration.
Testing the Epsilon Greedy Hypothesis
To test this hypothesis, add the code in Figure 2 to a new cell and execute it. This code creates the multi_armed_bandit function, which simulates a series of runs against a collection of slot machines. The function stores the observed odds of a jackpot payout. At each iteration, the agent will randomly play the slot machine with the best payout it has observed thus far, or arbitrarily try another machine. The argmax function returns the highest value in the numpy array. Here, that means the slot machine with the best odds of hitting a jackpot. The function’s parameters allow for control over the number of slot machines, the amount of iterations to run and the value of epsilon.
Figure 2 Reinforcement Learning Code
One Armed Bandit Slot Machine Odds Win
With the RL code in place, now it’s time to test the Epsilon Greedy algorithm. Enter the code from Figure 3 into an empty cell and execute it. The results show the chart from Figure 1 for easy reference, followed by the odds that the RL code observed.
Figure 3 Code to Compare the Actual Slot Machine Odds with the Agent’s Observations
As you can see in Figure 4, the algorithm did an excellent job, not only of determining the slot machine with the most favorable odds, but also producing fairly accurate payout probabilities for the other four slot machines. The graphs line up rather well. The exception being the fifth slot machine, which has such low odds of a payout that it scored negatively in the agent’s observations.
Figure 4 Results with an Epsilon Value of .1
Support:Email, Chat, Phone. White lotus casino no deposit bonus 2019. Withdraw time:1-3 days. Mobile.
Now, with the baseline established, it’s time to experiment some more. What would happen if epsilon were set to zero, meaning that the algorithm will never explore? Enter the following code in a new cell and execute it to run that experiment:
The resulting chart shows with one value higher than zero. One machine dominates the others, making it quite clear that the agent found one machine and stuck with it. However, run the code several times and you may notice that occasionally an interesting pattern develops. There will be one or more machines with negative values, with one machine with a higher than zero value. In these cases, the agent lost on a given machine and then won on another machine. Once the agent discovers a winning machine, it will stick with that machine, as it will be the machine that the argmax function will choose. If epsilon is set to zero, the agent may still explore, but it will not be intentional. As such, the observed slot machine odds are nowhere near the actual odds. It is also worth noting that the “greedy” method produces a lower reward score than when epsilon was set to .1. Greed, at least absolute greed, would appear to be counterproductive.
What if epsilon were set to 1, making the agent explore every time and not exploit at all? Enter the following code into a new cell and execute it:
The results will show that the agent did an excellent job of observing odds similar to those of the true odds, and the chart lines up very closely with Figure 1. In fact, the results of setting epsilon to 1 look very similar to when the value was .1. Take note of the Reward value, however, and there is a stark difference. The reward value when epsilon was set to .1 will nearly always be higher than when it’s set to 1. When the agent is set to only explore, it will try a machine at random at every iteration. While it may be learning from observation, it is not acting on those observations.
One Armed Bandit Slot Machine Odds Free Play
Wrapping Up
One Armed Bandit Slot Machine Odds Against
RL remains one of the most exciting spaces in artificial intelligence. In this article, I explored the Epsilon Greedy algorithm with the classic “Multi-Armed Bandit” problem, specifically drilling into the explore-or-exploit dilemma that agents face. I encourage you to further explore the trade offs by experimenting with different values of epsilon and larger amount of slot machines.
Frank La Vigneworks at Microsoft as an AI Technology Solutions professional where he helps companies achieve more by getting the most out of their data with analytics and AI. He also co-hosts the DataDriven podcast. He blogs regularly at FranksWorld.com and you can watch him on his YouTube channel, “Frank’s World TV” (FranksWorld.TV).
One Armed Bandit Slot Machine Odds Free
Thanks to the following technical expert for reviewing this article: Andy Leonard